CTSI Population Health promotes the use of AI as a transformational tool for health translation
An interview with CTSI's own Population Health Program
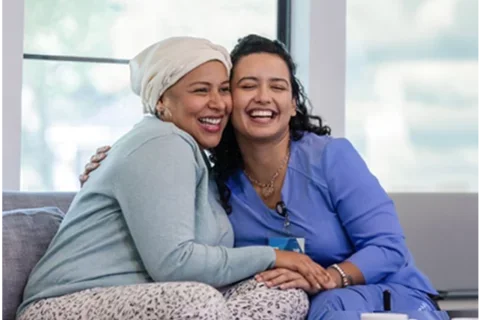
Imagine if all the groundbreaking medical research happening in labs could directly improve the health of everybody it’s intended for. The T1-T4 Translational Spectrum is a framework for describing this exact process. Knowledge progresses from basic science (T1) to our health care, social systems, and homes (T4). Clinical science begins at the T1 stage, Translation to Humans, which includes early-stage clinical trials and preclinical animal studies to test initial findings. T2, Translation to Patients, is when discoveries are then tested on humans in later phase clinical trials. In T3, Translation to Practice, research findings from T2 studies are introduced into medical practices. The T4 phase, Translation to Population Health, is concerned with making knowledge work in all places and times that it should, not just in studies, and not only for some people. T4 focuses on large scale application and the detailed work of making things work in the “last mile”, for all, thereby improving the health of entire populations. T4 translation is essential for making things work for everybody, and it is also important for sending feedback to earlier stages of translation regarding gaps in knowledge and things that are not working as hoped.
At the UCLA CTSI, the Population Health Program is developing innovative approaches to directly shape T4 translational science in a way that will have a lasting impact on communities throughout LA County. Hear from Moira Inkelas, PhD, MPH, M. Phil, Population Health Program Leader and TL1 Director, and Vladimir Manuel, MD, MS, Population Health Program Investigator, on some of the ways that Population Health is utilizing artificial intelligence (AI) as a tool for driving T4 translational research:
With regards to T4 translation, how would you describe the goals of the Population Health Program?
[Moira Inkelas] Population Health focuses on methods for T4 translation. We are better understanding AI as a transformational tool for T4 translation. It does something unique in terms of use of science at the bedside and curbside, as we often describe the last mile and moment of translation. It surfaces variation in care at the bedside/curbside that enables ongoing learning. Learning leads not only to optimal decision-making in the moment but permanently changes what clinicians do next time. The essence of the T4 phase is lasting change in how people think and what they do, informed by the best knowledge we have.
What are the potential applications of AI in T4 research?
[Vladimir Manuel] It is urgent that the scientific community understands this promise. So far, AI applications have largely focused on replacement functions. In other words, we substituting AI for what was previously done with human intelligence. There is a strong efficiency use case for repetitive and unnecessary human tasks. There is also a strong case for AI seeing patterns that are beyond what humans can detect.
The untapped promise of AI is T4 applications that enable humans to learn. They reveal patterns that cause reflection, they show specific situations that look alike but were handled differently, and they make time-intensive case reviews scalable (since currently, humans need to find illustrative cases).
This is of real interest to translational science institutes like our own, in part because private industry has yet to focus on and devote resources to developing AI within a learning framework. This robs health systems of untapped value and allows AI products with the wrong designs to proliferate. So many applications are called decision support but are actually replacement, without consideration of unique aspects of human intelligence. Using AI to enable learning is what some investigators in our Program are calling a second intelligence. The UCLA CTSI could lay the foundation for this area of AI, fostering growth in this area of translational science until market models find their way to it.
Tell us about the Program’s initial AI partnerships and successes.
[MI] Our first success is partnering with UCLA Health ISS (Information Services & Solutions, which maintains the central technology infrastructure for UCLA Health) and the UCLA Health command center, which is responsible for patient flow, to learn our way into this practice. ISS launched an AI Innovation Lab in Fall 2024 to foster use cases of AI-supported learning to improve practice, while having immediate impact on patients
The first prototype leverages a generative AI model to analyze historical task lists associated with patient transfers across UCLA Health facilities. This is a major pain point for health systems and patients alike. People who are in the wrong place for care, in a queue or boarding in the ED or unable to transfer into a quaternary care center, have poorer experiences and are at risk for safety events. The first prototype enables a model that identifies common features of patients who were successfully transferred from UCLA Health emergency departments to the three hospitals in our system. The initial use case will identify patients who share task list profiles similar to those who represent successful transfers in the past. This will flag them as potential transfer candidates in real time – which improves their care in the moment – and will provide learning opportunities for clinicians to improve their future practice. This first use case is underway and at the stage of identifying data elements.
From the patient perspective, how does this impact their care?
[VM] Most people are familiar with waiting longer than they feel they should, or bypassing where they wanted to go, such as to a nearby clinic, and going to the ED because it has the unscheduled access that they need. Patients may stay for hours or days in the ED because they are in a queue for a particular hospital, when another capable place has space for them. We are also aware that care can escalate in a place with gold standard care for any possibility. This leads to unwanted care, but is hard to intervene on once it begins. Variation in which suboptimal decisions or actions are not examined and learned from is unexamined inequity.
Have you experienced any hurdles to carrying out this project that you would like to make other researchers aware of?
[MI] This work requires methods that function in environments with multiple variables changing through time so lengthy fixed trials aren’t suitable. T4 methods study change in the reality in which the work takes place and the actual patients and communities in which the work takes place. Applying T1-T3 research methods can lead to the wrong conclusions, move to scale-up and implementation too early, and fall short of desired outcomes.
Learning frameworks aren’t afraid of complexity. The work won’t end in an automation or a standardizable conclusion. Instead, it’s a methodology and process that drives improvement.