Former CTSI TL1 fellow develops AI-powered tool to help detect rare diseases
Lead co-author, Dr. Katharina Schmolly, co-created the algorithm to detect cases of acute hepatic prophyria, a rare genetic condition
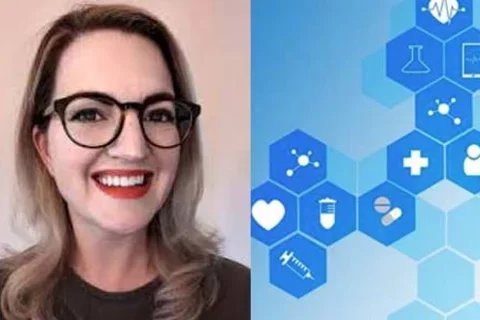
Several barriers to rare disease diagnosis make it difficult for patients to receive timely and appropriate treatment for their conditions. These barriers include a lack of treatment options, misdiagnoses of symptoms, and an overall general unawareness of rare conditions as a whole. To address this issue, researchers are turning to artificial intelligence and machine learning as powerful tools to provide potential solutions. Using AI-powered algorithms, doctors can comb through large data sets to find patterns and trends that can accelerate rare disease diagnosis.
"The diagnostic delay is roughly 10 to 15 years for these diseases because physicians don't see them very often,” said Dr. Katharina "Kat" Schmolly. “And while waiting for diagnosis, the disease can progress and cause irreversible damage. So, our goal is to diagnose patients earlier and manage their disease appropriately.”
While completing her CTSI TL1 Summer Fellowship as a medical student at the David Geffen School of Medicine, Dr. Schmolly began conducting research to do just that. Through her TL1 mentor, Dr. Simon Beavens, Dr. Schmolly partnered with a team of researchers at the University of San Francisco (UCSF) and Alnylam Pharmaceuticals to develop an algorithm to identify patients with acute hepatic porphyria (AHP), a rare genetic disease whose symptoms, which include, nausea, vomiting, limb weakness and bouts of chronic pain, overlap with more common diseases.
The multidisciplinary team made use of a rare and genetic disease database from the National Institutes of Health, along with de-identified electronic health care records (EHR) at UCLA and UCSF to create the algorithm to identify common patterns and symptoms to predict AHP in patients.
The research study, published in the Journal of the American Medical Informatics Association, found that the algorithm could predict patients who would be referred for AHP testing with 89 to 93 percent accuracy. In post hoc evaluations, they also found evidence that suggested that the study's machine learning models could identify 71% of AHP cases earlier than their diagnosis date, thus saving 1.2 years in diagnostic delays.
As a TL1 trainee, Dr. Schmolly utilized REDCap and CTSI Biomedical Informatics Program support for the EHR data extraction and sharing, in addition to CTSI Network Capacity Program support for recruitment and study administration. "This was a highly technical feat that could have not been achieved without the assistance and coordination services provided by the UCLA CTSI," said Dr. Schmolly.
Although there is still much work to be done in diagnosing and treating rare diseases, Dr. Schmolly is optimistic about the potential for the groundbreaking algorithm to change lives and improve care: "This study defines the first predictive model developed for rare disease, which we can now use as a template to create additional models for more rare diseases to directly improve the lives of patients--an example of truly translational research."
For more information on the study, read the recent press release in the UCLA Health Newsroom.
Image source: Handout photo, Katharina Schmolly, MD